Introduction
In the ever-evolving landscape of technology, quantum computing stands as a groundbreaking innovation with the potential to revolutionize various industries, including data science. As the world becomes increasingly data-driven, the demand for more powerful computing capabilities continues to rise. Quantum computing offers a glimpse into the future of computing, promising unprecedented processing power and the ability to solve complex problems that are currently beyond the reach of classical computers. In this article, we delve into the intersection of quantum computing and data science, exploring the frontiers of this exciting field.
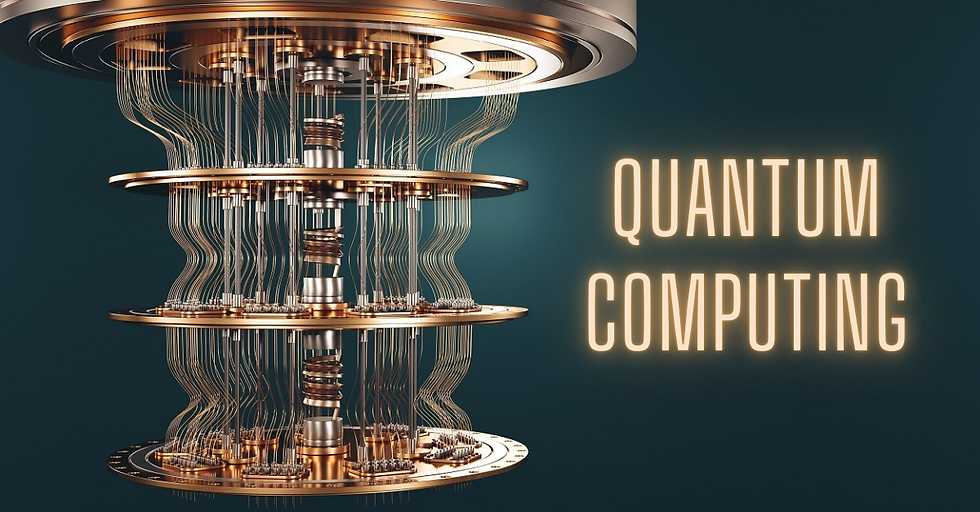
Understanding Quantum Computing:
Before delving into the implications for data science, it's essential to grasp the fundamentals of quantum computing. Unlike classical computers that use bits to represent information as either 0 or 1, quantum computers leverage quantum bits or qubits. Qubits can exist in multiple states simultaneously, thanks to the principles of superposition and entanglement, which are unique to quantum mechanics. This property allows quantum computers to perform calculations in parallel, offering exponential speedup for certain types of problems.
The Potential of Quantum Computing in Data Science:
Data science encompasses a wide range of activities, from data collection and storage to analysis and interpretation. Traditional data science techniques often face limitations when dealing with large datasets or solving complex optimization problems. Quantum computing has the potential to overcome these challenges by offering exponential speedup for certain types of calculations.
One area where quantum computing holds great promise is machine learning. Machine learning algorithms often involve training models on massive datasets, which can be time-consuming and computationally intensive. Quantum computers could accelerate this process significantly, enabling faster model training and more sophisticated algorithms. Moreover, quantum machine learning algorithms could unlock new possibilities, such as the ability to analyze high-dimensional data more efficiently or discover novel patterns that classical algorithms might overlook.
Another area where quantum computing could make a significant impact is in optimization problems. Many real-world problems, such as supply chain management, financial portfolio optimization, and drug discovery, can be formulated as optimization problems. Classical optimization algorithms often struggle to find optimal solutions in a reasonable amount of time, especially for large-scale problems. Quantum computing offers the potential to find solutions much faster by leveraging quantum parallelism and quantum optimization techniques such as quantum annealing and quantum approximate optimization algorithms (QAOA).
Challenges and Limitations:
While the potential of quantum computing in data science is undeniable, several challenges and limitations must be addressed before its widespread adoption. One major challenge is the current state of quantum hardware. Building and maintaining stable qubits with low error rates is incredibly challenging, and existing quantum computers are still relatively small and error-prone compared to their classical counterparts. Additionally, developing quantum algorithms that outperform classical ones for practical data science tasks remains an ongoing research challenge.
Another challenge is the need for quantum expertise. Quantum computing requires a fundamentally different approach to programming and algorithm design compared to classical computing. As such, there is a shortage of skilled professionals with expertise in both quantum mechanics and data science. Addressing this skills gap will be crucial for realizing the full potential of quantum computing in data science.
Despite these challenges, significant progress is being made in both quantum hardware and software development. Companies and research institutions around the world are investing heavily in quantum computing research, with the aim of overcoming current limitations and realizing the transformative potential of this technology.
Practical Applications:
While quantum computing is still in its infancy, there are already several practical applications in data science that could benefit from quantum algorithms. For example, quantum algorithms have been developed for tasks such as database search, pattern recognition, and molecular simulation, all of which are essential components of data science workflows.
In the field of finance, quantum computing could revolutionize portfolio optimization, risk analysis, and fraud detection by enabling faster and more accurate calculations. Similarly, in healthcare, quantum computing could accelerate drug discovery and personalized medicine by simulating complex biological systems with unprecedented precision.
Conclusion
The intersection of quantum computing and data science holds tremendous potential for driving innovation and solving some of the most pressing challenges facing society today. While there are still many hurdles to overcome, the rapid pace of progress in both quantum hardware and software development suggests that we are on the cusp of a new era in computing. By harnessing the power of quantum computing, we can unlock new insights, make better decisions, and ultimately, shape a brighter future for generations to come. Enroll now Data Science Training Institute in Gurgaon, Nashik, Kanpur, Dehradun, Kolkata, Agra, Delhi, Noida and all cities in India to be at the forefront of this transformative journey.
Comments