Introduction
In the landscape of data science, few technologies have made as profound an impact as deep learning. With its roots dating back to the 1940s, deep learning has experienced a renaissance in recent years, fueled by advancements in computational power, big data, and algorithmic innovation. This article aims to explore the intricate realm of deep learning, its applications across various industries, and its role in pushing the boundaries of data science.
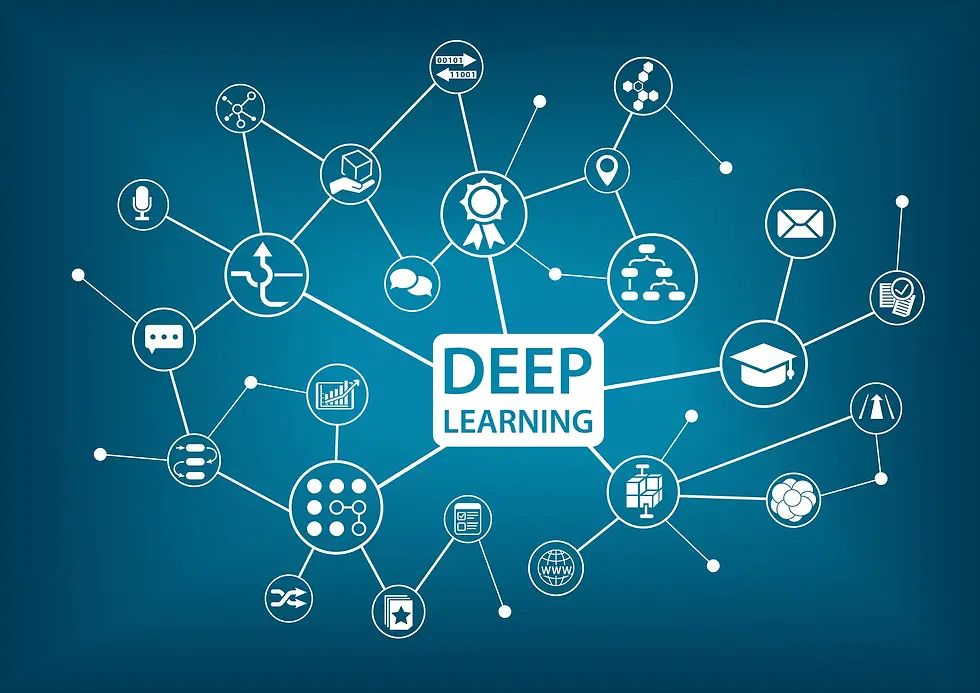
Understanding Deep Learning
At its core, deep learning is a subset of machine learning that involves training artificial neural networks to learn and make intelligent decisions from data. Unlike traditional machine learning algorithms that rely on handcrafted features, deep learning models autonomously discover intricate patterns and representations within the data, leading to more accurate predictions and insights.
Central to deep learning is the concept of neural networks, which are composed of layers of interconnected nodes called neurons. Each neuron applies a mathematical operation to its inputs and passes the result to the next layer. Through a process known as backpropagation, neural networks adjust their internal parameters (weights and biases) iteratively during training to minimize the difference between predicted and actual outputs.
Applications of Deep Learning
Deep learning has found applications across a myriad of domains, revolutionizing industries and pushing the boundaries of what is possible in data science. Some notable applications include:
Computer Vision: Deep learning has enabled remarkable progress in computer vision tasks such as object detection, image classification, and facial recognition. Convolutional Neural Networks (CNNs), a class of deep neural networks, have become the cornerstone of state-of-the-art vision systems, powering applications in autonomous vehicles, medical imaging, and surveillance.
Natural Language Processing (NLP): Deep learning has transformed the field of NLP, enabling machines to understand, generate, and translate human language with unprecedented accuracy. Models like Recurrent Neural Networks (RNNs) and Transformers have achieved remarkable results in tasks such as language translation, sentiment analysis, and text summarization, paving the way for applications in virtual assistants, chatbots, and content generation.
Healthcare: Deep learning holds immense potential in healthcare, where accurate diagnosis and treatment are paramount. Deep learning models have been deployed to analyze medical images, predict disease progression, and personalize treatment plans. From early detection of diseases like cancer to drug discovery and genomics, deep learning is reshaping the landscape of healthcare delivery and patient care.
Autonomous Systems: Deep learning plays a crucial role in enabling autonomy in various systems, including autonomous vehicles, drones, and robots. By leveraging deep learning algorithms for perception, decision-making, and control, these systems can navigate complex environments, recognize objects, and adapt to dynamic scenarios in real-time, unlocking new possibilities in transportation, logistics, and manufacturing.
Finance: In the financial sector, deep learning is employed for fraud detection, algorithmic trading, risk assessment, and customer service. Deep learning models can analyze vast volumes of financial data, identify patterns indicative of fraudulent activities, and make real-time trading decisions based on market trends and signals, enhancing efficiency and mitigating risks in financial operations.
Challenges and Future Directions
Despite its remarkable achievements, deep learning is not without its challenges. Training deep neural networks requires substantial computational resources and labeled data, posing barriers to entry for smaller organizations and researchers. Moreover, deep learning models often lack transparency and interpretability, raising concerns about bias, ethics, and accountability in AI systems.
Looking ahead, researchers are actively exploring avenues to address these challenges and unlock the full potential of deep learning. Techniques such as transfer learning, semi-supervised learning, and reinforcement learning hold promise for overcoming data scarcity and improving model generalization. Additionally, efforts to enhance interpretability and fairness in deep learning models are gaining traction, with the aim of fostering trust and accountability in AI systems.
Conclusion
Deep learning stands at the forefront of the data science revolution, driving innovation across industries and pushing the boundaries of what is achievable with data. From revolutionizing healthcare and finance to enabling autonomous systems and transforming how we interact with technology, the impact of deep learning is profound and far-reaching. As we continue to harness the power of deep learning, it is imperative to address its challenges and ethical implications, ensuring that AI technologies are developed and deployed responsibly for the benefit of society. Enroll now at the Best Data science Training in Gurgaon, Kanpur, Dehradun, Kolkata, Agra, Delhi, Noida and all cities in India.
Comments